Hi! I'm Nihaal
This is a brief overview of my education, projects, skills and background
Feel free to reach out to me for opportunities, collaborations, or any discussions related to my areas of interest
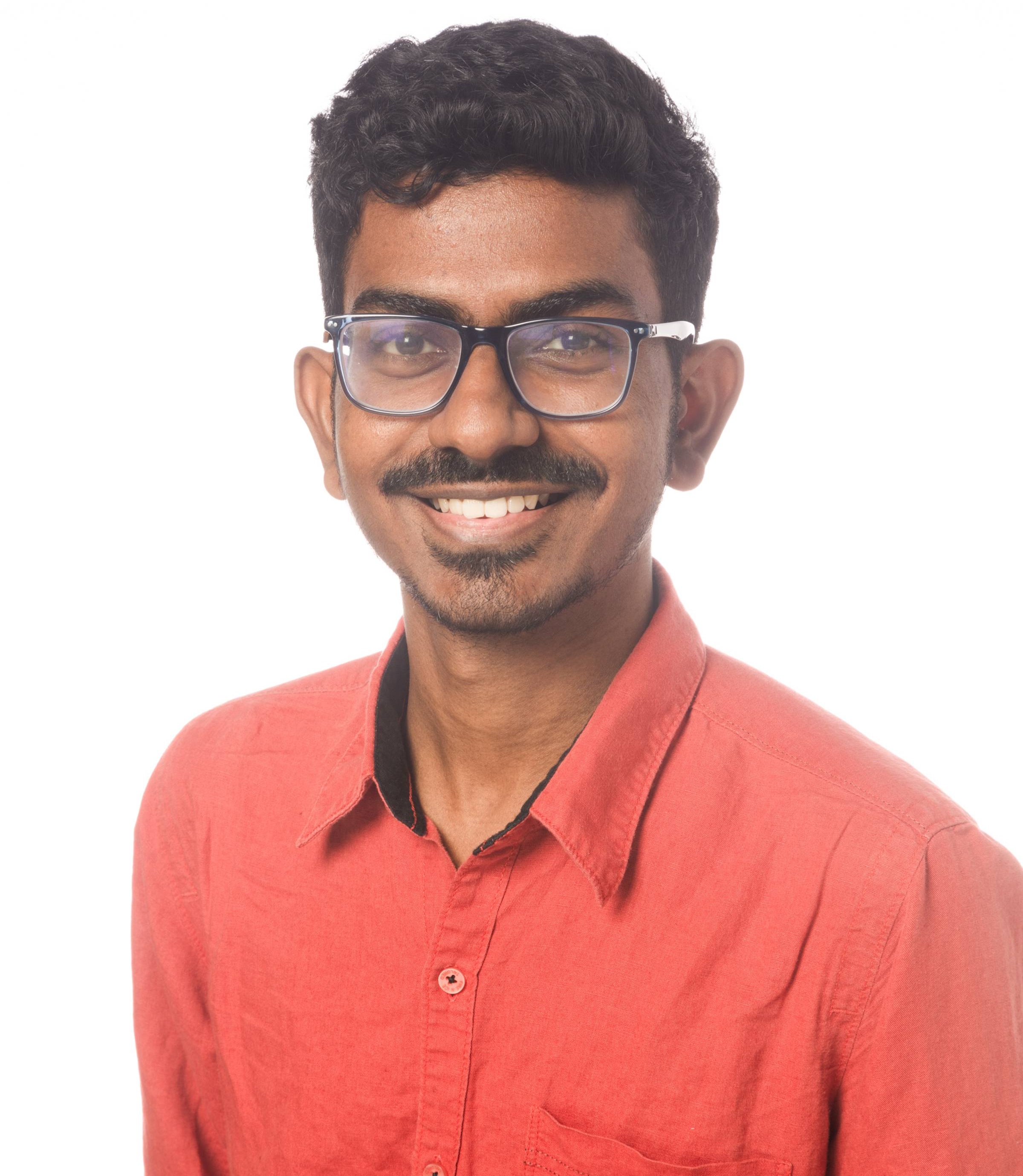
- Designed a deep learning-based web application to generate captions for input images
- Based on an encoder-decoder model and transfer learning
- Used Inception V3 for image feature extraction and an LSTM with GloVe embeddings for caption generation
- Implemented in python using Tensorflow-Keras and NLTK and deployed on the web using Angular for frontend and Flask as backend
- Work published in the International Journal of Information Technology and Electrical Engineering
- Designed a photo-sharing web app inspired by Instagram
- Focused on maximizing accessibility for disabled users
- Enabled users to create accounts, engage with posts, and connect with others accounts
- Implemented using JavaScript, React, Flask, REST, Heroku and PostgreSQL
- Designed a web interface to create TED Talks-style infotainment videos from user-generated prompts
- Fine-tuned the T5 Text-to-Text LLM for script generation and web scraped content from Wikipedia
- Designed a predictive model to detect automated paraphrasing for plagiarism evasion
- Used sentence embeddings generated by the SentenceBERT and MPNet models
- Achieved a peak accuracy of 89.00%, surpassing the previous state-of-the-art result of 83.36%
- Additionally, designed a smaller, efficient variant of the model that utilized only the first three sentences of the input paragraph, yet achieved an accuracy of 87.64%
- Designed a contextual AI assistant that parses recipes from allrecipes.com and guides the user through making the recipes in the form of a conversational interface
- Understands navigation utterances, context-based questions and answers user queries
- Implemented in python using RASA, NLTK and Spacy
- Fine-tuned the BERT language model on the OpenBookQA dataset for multiple-choice question answering
- Achieved a 3% improvement over baseline performance by pre-training on the CommonSenseQA dataset
- Analyzed the impact of various medical conditions and additional factors on COVID-19 severity and mortality rates
- Developed and implemented diverse classification models, including Neural Networks, KNNs, Weighted KNNs, Naïve Bayes Classifiers, Decision Trees, and Random Forest
- Evaluated and compared the performance of each model in predicting COVID-19 severity and fatality rates, assessing their relative effectiveness through comprehensive performance metrics
- Conducted hypothesis testing using “Student's T-test” to verify the significance of findings
- Analyzed an extensive dataset of 1.88 million wildfires, incorporating 50 distinct features
- Performed EDA using Tableau for data visualization and OpenRefine for data cleaning and imputation
- Implemented feature engineering using one-hot encoding and min-max scaling through Pandas and Sklearn
- Developed models for predicting the cause of the fire, estimating the size of the fire, and determining whether arson was involved using Histogram-based Gradient Boosting Classification Trees
- Achieved accuracies of 61.8%, 75.1%, and 89.2%, respectively